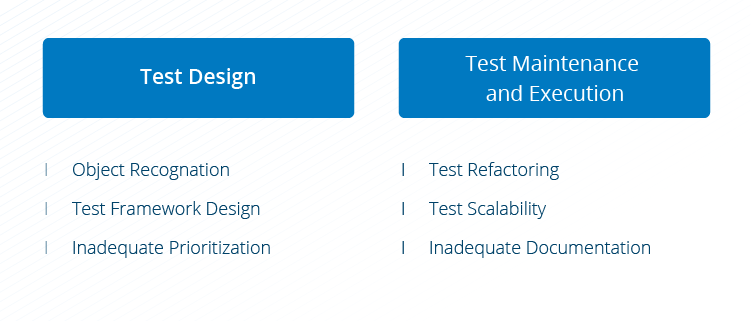
Transfer learning can help businesses adapt and thrive in a changing workforce. This involves applying machine learning algorithms in order to identify subjects and their contexts. These algorithms can be retained in their entirety, making it less difficult to recreate them. Here are some tips for applying transfer learning to businesses:
Techniques
Transfer learning is a process that allows machine learning models to learn from the same or similar data. Natural language processing, for instance, can use models that can recognize English speech to detect German speech. An autonomous vehicle can use a model designed for driverless cars to recognize different types of objects. Transfer learning, even if the target language may be different, can improve the performance and efficiency of machine learning algorithms.
One common technique is called "deep transfer learning." This method generally teaches the same or similar tasks across different datasets. The technique allows neural network to learn quickly and easily using previous experiences, thus reducing training time. Transfer learning algorithms are more precise and require less effort than creating new models. Researchers are increasingly exploring the potential benefits of transfer-learning, as it has become increasingly popular.
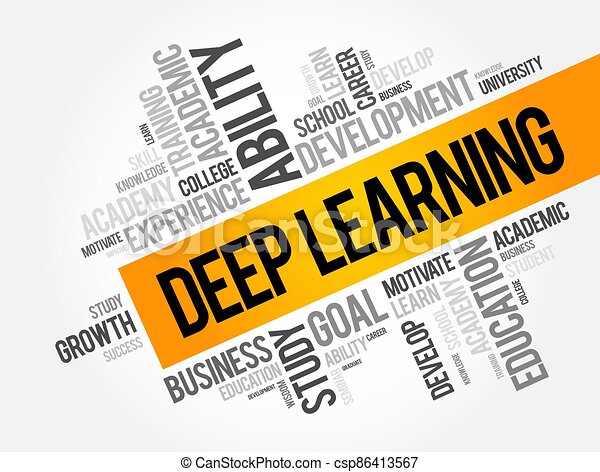
Tradeoffs
Transfer learning can be described as a cognitive process in the which a learner brings together knowledge from different domains. The process of transfer learning involves observation in the target domain and knowledge from the source domain. Similar strategies are used when building the model. The method has its own tradeoffs. This article will talk about the tradeoffs you can make with different learning environments. Learn how to evaluate different learning strategies for transfer.
Transfer learning has the major disadvantage that it reduces the performance of the model. Negative Transfer occurs when the model has been trained on large amounts of training data and is not able to perform well within the target domain. Another downside to transfer learning is the possibility of overfitting. This is a problem in machine learning because the model learns too much from the training data. Therefore, transfer learning is not always the best approach for natural language processing.
Indications of effectiveness
Transfer learning is a wonderful way to create and train neural networks in many different domains. Transfer learning can be applied to empirical programming engineering, which requires large labeled datasets. It is also useful for practitioners to create deep architectures, without the need to customize. Although the evidence of transfer learning's effectiveness is varied, they all point towards a successful outcome. Here are three.
Comparing the performance of models across different datasets has allowed us to evaluate their effectiveness. We have had varying degrees success. When differences between datasets are large, transfer is more effective than unsupervised learning. Hence, the two methods are recommended for large datasets. Transfer learning has many performance metrics, including accuracy and specificity. This article will present the main findings from supervised learning and transferred learning.
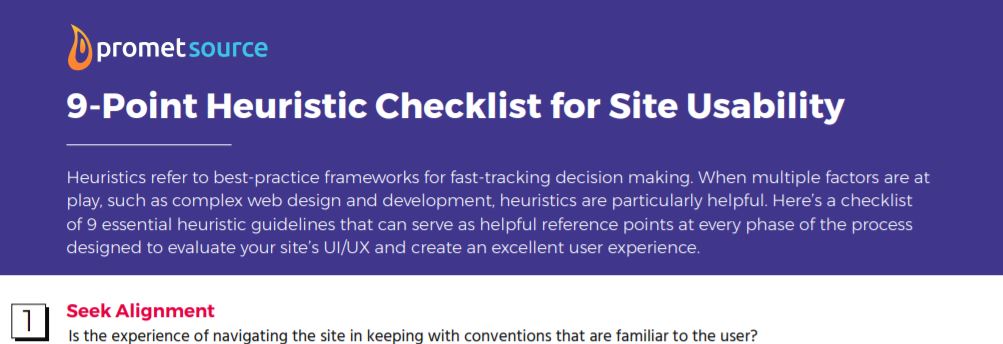
Applications
Transfer learning is when a model is transferred from one task to another. For example, a model developed to detect car dings could be used in detecting buses, bikes and even chess. This knowledge transfer is particularly helpful for ML tasks that require models with similar physical properties. In addition, it has the potential to improve the performance of machine-learning systems. But what are the applications of transfer learning? Let's talk about some.
NLP is one of the most common applications of transfer learning. It leverages existing AI models and is therefore a key advantage. In this way, the system can learn to optimize conditional probabilities of certain outcomes in textual analysis. One of the most common problems in sequence labeling is taking text as input and predicting an output sequence containing named entities. These entities can be recognized, and then classified using word-level representations. This process can be dramatically shortened using transfer learning.
FAQ
What are some examples AI-related applications?
AI is used in many areas, including finance, healthcare, manufacturing, transportation, energy, education, government, law enforcement, and defense. These are just a handful of examples.
-
Finance – AI is already helping banks detect fraud. AI can scan millions of transactions every day and flag suspicious activity.
-
Healthcare - AI can be used to spot cancerous cells and diagnose diseases.
-
Manufacturing - AI can be used in factories to increase efficiency and lower costs.
-
Transportation - Self-driving vehicles have been successfully tested in California. They are now being trialed across the world.
-
Utility companies use AI to monitor energy usage patterns.
-
Education – AI is being used to educate. Students can, for example, interact with robots using their smartphones.
-
Government - AI can be used within government to track terrorists, criminals, or missing people.
-
Law Enforcement-Ai is being used to assist police investigations. Detectives can search databases containing thousands of hours of CCTV footage.
-
Defense – AI can be used both offensively as well as defensively. An AI system can be used to hack into enemy systems. Defensively, AI can be used to protect military bases against cyber attacks.
How will governments regulate AI?
While governments are already responsible for AI regulation, they must do so better. They must ensure that individuals have control over how their data is used. Companies shouldn't use AI to obstruct their rights.
They should also make sure we aren't creating an unfair playing ground between different types businesses. Small business owners who want to use AI for their business should be allowed to do this without restrictions from large companies.
What is the future role of AI?
The future of artificial intelligent (AI), however, is not in creating machines that are smarter then us, but in creating systems which learn from experience and improve over time.
We need machines that can learn.
This would allow for the development of algorithms that can teach one another by example.
We should also look into the possibility to design our own learning algorithm.
It's important that they can be flexible enough for any situation.
Who is the leader in AI today?
Artificial Intelligence (AI), a subfield of computer science, focuses on the creation of intelligent machines that can perform tasks normally required by human intelligence. This includes speech recognition, translation, visual perceptual perception, reasoning, planning and learning.
Today there are many types and varieties of artificial intelligence technologies.
The question of whether AI can truly comprehend human thinking has been the subject of much debate. Recent advances in deep learning have allowed programs to be created that are capable of performing specific tasks.
Google's DeepMind unit, one of the largest developers of AI software in the world, is today. It was founded in 2010 by Demis Hassabis, previously the head of neuroscience at University College London. In 2014, DeepMind created AlphaGo, a program designed to play Go against a top professional player.
Statistics
- The company's AI team trained an image recognition model to 85 percent accuracy using billions of public Instagram photos tagged with hashtags. (builtin.com)
- Additionally, keeping in mind the current crisis, the AI is designed in a manner where it reduces the carbon footprint by 20-40%. (analyticsinsight.net)
- That's as many of us that have been in that AI space would say, it's about 70 or 80 percent of the work. (finra.org)
- By using BrainBox AI, commercial buildings can reduce total energy costs by 25% and improves occupant comfort by 60%. (analyticsinsight.net)
- A 2021 Pew Research survey revealed that 37 percent of respondents who are more concerned than excited about AI had concerns including job loss, privacy, and AI's potential to “surpass human skills.” (builtin.com)
External Links
How To
How to create an AI program that is simple
It is necessary to learn how to code to create simple AI programs. There are many programming languages out there, but Python is the most popular. You can also find free online resources such as YouTube videos or courses.
Here is a quick tutorial about how to create a basic project called "Hello World".
You'll first need to open a brand new file. You can do this by pressing Ctrl+N for Windows and Command+N for Macs.
Then type hello world into the box. To save the file, press Enter.
For the program to run, press F5
The program should show Hello World!
This is just the start. These tutorials will help you create a more complex program.